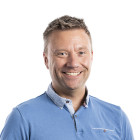
Graham Leonard Natural Hazard and Risk Theme Leader
Graham is a Principal Scientist within the Earth Structure and Processes Department. His particular research interests are in Taupo Volcanic Zone volcanic mapping; New Zealand volcanic geology, stratigraphy and geochronology; developing effective response to warning systems, especially for volcanic, tsunami & landslide/debris-flow processes; and quantifying/characterising & mitigating the impacts of natural hazard events.
View Bio Contact Me